The Best Fit
DistributionProbe™
Probability Distribution Models
Historically, the decision process has been fundamentally deterministic in nature. Put another way, there has been an assumption that all pertinent decision variables are basically without variation. Uncertainties in the knowledge of the specifics of such variables are dealt with indirectly using arbitrary applied safety factors (e.g., the addition of a 30% safety factor to past estimates). Thus, decisions based on the use of safety factors can have probabilities of failure that may vary from overly conservative low values to unacceptably high values, depending on the actual uncertainties and variations associated with pertinent variables. In either case, this approach is sure to have a significant negative impact on your bottom line.
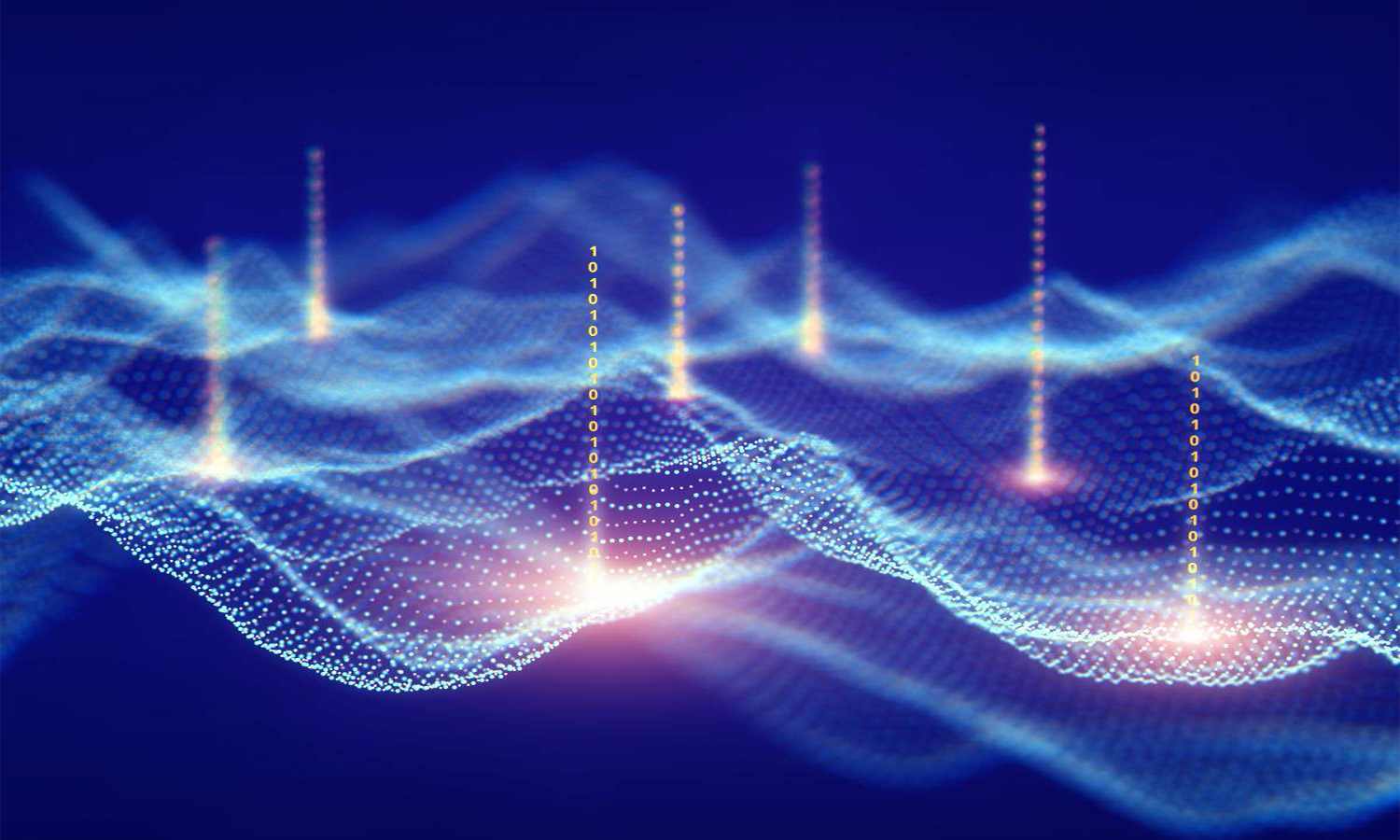
In an increasingly competitive marketplace, today’s companies should be very uncomfortable with such an uncertain approach to decision-making and the often unexpected and damaging results. This is particularly true in fields involving new or advanced applications of technology, where the demand for higher competitiveness requires increasing emphasis on accuracy and realism in analytic modeling and decision process. The required accuracy and realism can only be obtained by adequately accounting for uncertainty, usually by characterizing pertinent variables as random variables, which can only be defined by probability distribution models.
Delivered to the desktop through a familiar Windows-based interface, DistributionProbe identifies the best probability distribution model for such variables and uncertainties using available data. The large library of distribution type, appropriate methods for fitting the distributions to your small to large number of datapoint, and objective and subjective techniques for verification and validation of fitted distribution types assure accuracy of variable models.
Proprietary Process
Our proprietary processes will easily guide you to construct probability distribution models for your process variables when no data is available. Additionally, the Bayesian updating capability of our SPISE Platform enables you to further customize and improve the accuracy of the any probability distribution when new data becomes available.
DistributionProbe can be used as a standalone tool or used together with SPISE and/or UNIPASS Software. Some of the key capabilities of DistributionProbe include:
- 15 probability distributions to identify the best fit for your data.
- Three methods to estimate distribution parameters including the maximum likelihood method, method of moments, and modified method of moments.
- Three goodness-of-fit tests including Kolmogorov-Smirnov (K-S) test, Anderson-Darling test and Cramer Von Mises test to objectively determine the best-fit distribution among the available distributions.
- Probability Plot (Probability Paper) to evaluate the selected distributions subjectively.
- Two calculators: one for calculating goodness-of-fit tests critical values and one for computing probability density function (PDF) and cumulative probability function (CDF) values.
A comprehensive HTML format report containing all pertinent information including, but not limited to, full comparison between various distributions, distribution parameters, PDF and CDF plots for all selected distributions, probability plots, and more
Learn More
Connect with us for a demonstration on how Predictive Technology can take the stress and strain out of your most rigorous business dilemmas.
Learn More
Connect with us for a demonstration on how Predictive Technology can take the stress and strain out of your most rigorous business dilemmas.
Related Solutions
Tough Times Require Smart Decisions
In these pandemic times, businesses need to be creative and resourceful with less. Our business solutions help you do more than ever all while protecting your valuable resources. We may have the solution you have been looking for. Schedule a consultation with one of our professionals to get started.